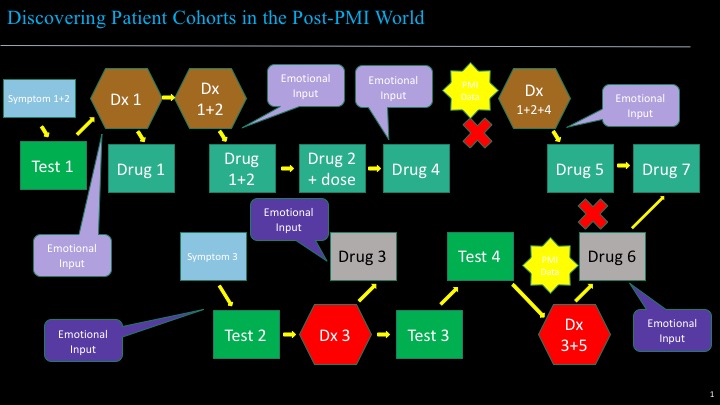
Complex patient analysis – discovering the patient cohorts that make the difference (part 3)
Patients are very complex things to analyse and unfortunately most of our analyses don’t touch on the true complexities.
We get in our own way much of the time because we think that we understand a patient population because we can define them. This so called “Defined Cohort” drives all our clinical research as well as almost all our market research. We define the cohort we want to explore, often ignoring confounding factors – but it is often those very same confounding factors that are what we should be aiming to research in the first place.
We’re familiar with the cohorts that we find in research that result from some differences we found in the research. In clinical trials this is often response vs non-response; in market research, it is respondents who like the concept vs those that don’t – but still the core research is based on the predefined cohort we started with.
What we most frequently miss are the cohorts that we don’t know to research because we didn’t go looking for them in the first place – I call these the “Found Cohorts”. Just like the Hubble telescope looking at empty sky and finding galaxies, so too we can use data exploration methods to find the hidden cohorts in our data. I’m not talking about finding cohorts for the sake of it, but finding cohorts that have real clinical significance.
Take complex co-morbidities as an example. If you start looking at a closed patient population with a closed analytical view you will find the co-morbidities that you expect. If you expand your view to include more complete data (using EHR or claims data, for instance) you can often find cohorts within your defined target group that behave very differently due to factors that you hadn’t previously considered
- high levels of anxiety and/or depression in COPD
- potential links between a surgical procedure and a chronic condition in diabetes
- unexpected disease prevalence due to living conditions in a developing country.
Co-morbidity is a single dimension of complexity, and recent studies have shown us that the entire flow of patient management shows many layers that add clarity. This makes a huge difference to our understanding. Patients go through many stages of diagnosis and treatment. Often they have overlapping conditions. Through all the different stages of diagnosis and treatment the patients’ needs change. These changes need to be characterized and measured.
In these circumstances of improved understanding a range of opportunities become clear:
- Product communication or positioning opportunities due to weaknesses in the competition
- Groups of under-treated patients that can be reached once they are understood
- Sales force retargeting because treatment potential can be better defined
The data and analytical tools exist to generate these types of insight. The effect can be most illuminating. Contact us for more details, a demonstration, or just a discussion.